Guest Essay
Biological Variation Data for setting Quality Specifications
Dr. Callum G. Fraser, one of the leading experts in defining quality goals, has provided us with an article based on his presentation at the 1998 Fourth European Conference Quality [r]evolution in Clinical Laboratories in Antwerp. Dr. Fraser provides a detailed discussion of biologic goals and describes the process as well as the sources of data. Links in the essay are provided to tables on this webiste that contain the data.
Directorate of Biochemical Medicine,
Ninewells Hospital and Medical School,
Dundee DD1 9SY Scotland
- Introduction: Setting Quality Specifications
- The Nature of Biological Variation
- Generation of Data on Biological Variation
- Monitoring Individual Patients
- Diagnosis
- Other Performance Characteristics
- Conclusions
- References
- Biographical details - Callum G. Fraser
Introduction - setting quality specifications
The many needs for quality specifications in laboratory medicine have been discussed in detail previously.[1] For quality planning, using the many techniques and tools in this website, quality specifications are a necessary prerequisite. It is often suggested that quality specifications should be based upon "medical needs". Certain quality specifications, particularly for practicability characteristics such as turnaround time and sample type and volume, could be obtained by discussion with clinicians, circulation of questionnaires or analysis of feedback from users. However, such strategies may not be the most appropriate to generate quality specifications for precision and bias. Per Hyltoft Petersen, in a past Guest Essay [June 1997], [2] has detailed some European approaches to analytical goal-setting based on biology. This Essay, based upon a presentation at the 1998 Fourth European Conference on Quality [R]evolution in Clinical Laboratories [Antwerp, Belgium, 29/30 October 1998] will discuss the generation of data on biological variation and demonstrate that quality specifications derived from these are indeed firmly based upon the major clinical uses of test results, namely, monitoring and diagnosis. This essay also provides a link to a data bank on biological variation that was recently published by Sebastian-Gambaro, Liron-Hernandez, and Fuentes-Arderiu [3]. Access to this data bank should allow much wider use of biological variation for defining quality specifications.
The nature of biological variation [4]
Many of the quantities measured in laboratory medicine change over the span of life: one repercussion is that population-based reference values may need to be stratified into sub-groups according to age. A small number of quantities vary in predictable cyclical rhythms which may be daily, monthly, or seasonal in nature. Knowledge of these rhythms is important because it would be very difficult to create population-based reference values for each and every point in a cycle: this means that the appropriate times to investigate a cycle must be defined and reference values generated for these. Moreover, absence of predicted rhythms may give indications to the presence of disease.
However, most quantities do not have predictable cyclical rhythms. It can be considered that, for each individual, the quantity varies round a homeostatic set point in a truly random manner: Table 1 shows the serum creatinine concentrations [umol/L] in four ostensibly healthy individuals in six samples taken at daily intervals [taken from Fraser CG, Stevenson HP. Production and use of data on biological variation in laboratory medicine. CPD Bulletin: Clinical Biochemistry 1998;1:5-8, with permission of Rila Publications Ltd., London].
Table I: Serum creatinine [umol/L] in four apparently health individuals in six samples taken at daily intervals
It is obvious that each of the four subjects has different mean values [set points]. There are clearly large differences among the set points of individuals and this is termed between-subject biological variation. Moreover, each subject varies in a random manner around their own homeostatic set-point - this fluctuation is due to three factors, namely, pre-analytical variation, random analytical variation [precision and changes in bias usually measured as precision] and inherent within-subject biological variation. It is important for the setting of general quality specifications to appreciate that different quantities have different magnitudes of within- and between-subject biological variation. Table 2 shows the serum iron concentrations [umol/L] in four apparently healthy individuals in six samples taken at daily intervals [taken from Fraser CG, Stevenson HP. Production and use of data on biological variation in laboratory medicine. CPD Bulletin: Clinical Biochemistry 1998;1:5-8, with permission of Rila Publications Ltd., London].
Table 2: Serum iron [umol/L] in four apparently health individuals in six samples taken at daily intervals
Creatinine has small within-subject biological variation and large between-subject variation whereas, in contrast, iron has large within-subject biological variation and small between-subject variation; these differences are shown visually in Figure 1.

Figure 1
Mean and absolute ranges for serum creatinine [upper panel] and serum iron [lower panel] showing quantities with high and low individuality [low and high indices of individuality] respectively [taken from Fraser CG, Stevenson HP. Production and use of data on biological variation in laboratory medicine. CPD Bulletin: Clinical Biochemistry 1998;1:5-8, with permission of Rila Publications Ltd., London].
Generation of data on biological variation
It is reasonably easy to generate data on within- and between-subject biological variation and this has been described in detail previously. [5] The strategy is akin to the generation of population-based reference values [6] but that more than one sample is obtained from each of a small cohort of subjects rather than a single sample being obtained from a large number of subjects.
Subjects are usually chosen from the easily available reference population, normally ambulant ostensibly healthy members of laboratory or hospital staff, or students. Before samples are collected, the many pre-analytical sources of variation are minimised as far as possible so that they do not confound the estimates of within-subject biological variation. Then, a small series of samples is collected at appropriate times from each subject. All of the samples are handled and treated according to strict standard protocols and then stored to ensure stability. Finally, the quantities are measured in duplicate. This strategy of analysis in replicate is adopted to ensure that the analytical random variation can be obtained from native samples thus ensuring appropriate concentrations and matrix. Analysis of the samples is best done so as to minimise analytical variation by using a single analyst and one analytical method with, ideally, single lots of reagents, calibrators, and consumables. If the quantity is unstable, then clearly analysis needs to be done at the time of collection and, here, stable quality control materials must be assayed in each batch to be able to calculate the analytical component of the variation. Then, the presence of outliers at each information stratum is evaluated and nested analysis of variance applied to calculate analytical variation [CVA], within-subject variation [CVI], and between-subject variation [CVG].
Monitoring individual patients
Changes in serial results can be due to -
- the patient getting better,
- the patient getting worse,
- pre-analytical variation,
- biological variation and
- analytical variation - changes in bias and inherent precision.
Thus, if pre-analytical sources of variation are minimised by good phlebotomy and standard sample transport, handling and storage techniques, then, to assess whether a patient has got better or worse, then the change must exceed the inherent variation due to biological and analytical variation. The reference change value [RCV] must exceed -
where Z is the number of standard deviates appropriate to the probability selected [for example, 1.96 for P < 0.05 and 2.56 for P < 0.01].
It is clear that, in consideration of the effect of analytical precision in the medical needs context of monitoring change in an individual patient, attention to biological variation is mandatory and it is within-subject variation that is important. The amount of variability added to true test result variability [biological] by analysis [precision] can easily be calculated and a graphical representation of the variability added when CVA is different fractions of CVI is shown in Figure 2.[7]
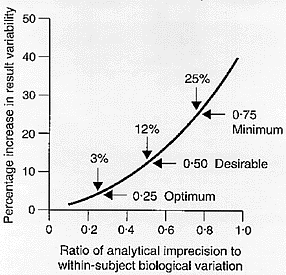
Figure 2
Percentage increase in test result variability due to analytical precision [expressed as a ratio of analytical to within-subject biological variation] showing three possible quality specifications based on within-subject biological variation. From Fraser CG, Hyltoft Petersen P, Libeer JC, Ricos C. Proposals for setting generally applicable quality goals solely based on biology. Ann Clin Biochem 1997;34:8-12, with permission.
As CVA rises, the amount of added error rises - and this rise is not simply linear. The concept that analytical variation should be less than one-half the average within-subject biological variation was first proposed by Cotlove et al. [8] The statistical basis was elaborated by Harris [9] who demonstrated that, if the analytical coefficient of variation [CVA] was less than one-half the average within-subject variation [CVI], that is CVA < 0.5CVI , then the amount of variability added was about 10% [in reality, 11.8%] which was stated to be "reasonable".
This concept was recently expanded.[7] Increasing the analytical precision relative to the within-subject biological variation increases the test result variability. More detailed numerical analysis showed that when CVA < 0.75CVI then at most 25% variability is added to test result variability, when CVA < 0.50CVI then less than 12% variability is added and, when CVA < 0.25CVI then a maximum of 3% variability is added. In consequence, we proposed, as shown in Figure 2, that:
desirable performance was defined by CVA < 0.50CVI and that the quality specifications generated should be viewed as being generally applicable, but that users of quality specifications based on biology might consider that
optimum performance could be defined by CVA < 0.25CVI and that the more stringent quality specifications generated using this formula should be used for those quantities for which the desirable performance standards were easily achieved with current technology and methodology, and that
minimum performance was defined by CVA < 0.75CVI and that the less stringent quality specifications generated using this formula should be used for those quantities for which the desirable performance standards were not attainable with current technology and methodology.
Diagnosis
Although test results can be compared against fixed criteria such as those based upon risk [cholesterol], consensus clinical guidelines [glucose] or locally agreed algorithms, most diagnostic decisions seem to based upon comparison with population-based reference limits.
The dispersion of the reference interval is made up of analytical variation, within-subject biological variation and between-subject biological variation. If the analytical variation was negligible, then the dispersion would be equivalent to 1.96 [CVI2 + CVG2]0.5 - that is, 1.96 times the "group" biological variation.
The percentage increase in the population outside each reference limit as a function of the ratio of the bias to the group biological variation is shown in Figure 3.[7] A positive bias will increase the percentage outside the upper reference limit and decrease the percentage outside the lower reference limit. A negative bias will have identical effects but on the opposite reference limits. The concept that the ideal was that, from a medical needs point of view, laboratories throughout a homogeneous population area should use exactly the same reference intervals was first proposed by Gowans et al.[10] who showed that, for this to be achieved, bias [BA], should be
It can be calculated that when [BA] < 0.375[CVI2 + CVG2]0.5 then 1.0% are outside one reference limit and 5.7% outside the other, when [BA] < 0.250[CVI2 + CVG2]0.5 then 1.4% are outside one reference limit and 4.4% outside the other and when [BA] < 0.125[CVI2 + CVG2]0.5 then 1.8% are outside one reference limit and 3.3% outside the other. In consequence, analogously to precision above, we recently considered, as shown in Figure 3,[7] that:
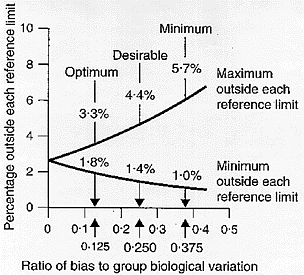
Figure 3
Percentage increase in test result variability due to analytical bias [expressed as a ratio of analytical to group (within- plus between-subject) biological variation] showing three possible quality specifications based on group biological variation. From Fraser CG, Hyltoft Petersen P, Libeer JC, Ricos C. Proposals for setting generally applicable quality goals solely based on biology. Ann Clin Biochem 1997;34:8-12, with permission.
desirable performance was defined by [BA] < 0.250[CVI2 + CVG2]0.5 and that the quality specifications generated should be viewed as those generally applicable, but that users of quality specifications based on biology might consider that
optimum performance was defined by [BA] < 0.125[CVI2 + CVG2]0.5 and that the more stringent quality specifications generated using this formula should be used for those quantities for which the desirable performance standards are easily achieved with current technology and methodology, and that
minimum performance was defined by [BA] < 0.375[CVI2 + CVG2]0.5 and that the less stringent quality specifications generated using this formula should be used for those quantities for which the desirable performance standards are not attainable with current technology and methodology.
Other performance characteristics.
As outlined previously by Hyltoft Petersen,[2] the current European consensus is clearly that precision < 0.5CVI, and that bias < 0.250[CVI2 + CVG2]0.5 . Moreover, quality specifications for other performance characteristics can also be based on biology.
Quality specifications can be set for the allowable difference between two methods used for analysis of the same quantity in a single laboratory as:[11]
Quality specifications for drugs can be calculated using a model similar to that based on biological variation[12] but using simple pharmacokinetic theory as:
where T is the dosing interval and t the half-life.
Biological variation data can be used to set specifications for the fixed limits of acceptance in all external quality assessment schemes,[13] that is [for P < 0.01]:
which is a simple combination of the desirable quality specifications for precision and bias.
It has been suggested that when reference methods are used for the validation of routine methods, the more stringent of the quality specifications proposed above be used but that, for this application, they be halved. When such methods are used to set values for use in EQA schemes, the fixed limits of acceptance [for P < 0.01] should be divided by a factor of five.[14]
Conclusions.
Thus, expert European professional consensus is that quality specifications are best based on calculations involving biological variation. These strategies have advantages in that they are directly related to medical needs. Moreover, data on components of biological variation are available on many quantities and there are a number of compilations in the easily available literature. A recent publication which follows this Essay[3] makes the data now very easy to obtain. Further, the estimates seem generally independent of study location, number of subjects, length of study, analytical methodology, age of subjects or whether they are in a state of health or have stable but chronic disease. The published data can therefore be used ubiquitously and there is no need for laboratories to derive their own. It is highly likely that further databases will be published in the future since data on the components of biological variation keep on being published [mainly in Clinical Chemistry, Clinical Chemistry and Laboratory Medicine, Annals of Clinical Biochemistry and Scandinavian Journal of Clinical and Laboratory Medicine, but also in many other more specialist journals]. It is hoped that these will become available through electronic means.
References
- Fraser CG. Quality specifications in laboratory medicine. Clin Biochem Revs 1996;17:109-14.
- Hyltoft Petersen P. Some European approaches to analytical goal-setting.
- Sebastian-Gambaro MA, Liron-Hernandez FJ, Fuentes-Arderiu X. Intra- and inter-individual biological variability data bank. Eur J Clin Chem Clin Biochem 1997;35:845-52.
- Fraser CG. Interpretation of clinical chemistry laboratory data. Oxford 1986, Blackwell Scientific, 179pp.
- Fraser CG, Harris EK. Generation and application of data on biological variation in clinical chemistry. Crit Rev Clin Lab Sci 1989;27:409-437.
- Solberg HE, Grasbeck R. Reference values. Adv Clin Chem 1989;27:1-79.
- Fraser CG, Hyltoft Petersen P, Libeer JC, Ricos C. Proposals for setting generally applicable quality goals solely based on biology. Ann Clin Biochem 1997;34:8-12.
- Cotlove E, Harris EK, Williams GZ. Biological and analytic components of variation in long-term studies of serum constituents in normal subjects. III. Physiological and medical implications. Clin Chem 1970;16:1028-32.
- Harris EK. Statistical principles underlying analytic goal-setting in clinical chemistry. Am J Clin Pathol 1979; 374:72-82.
- Gowans EMS, Hyltoft Petersen P, Blaabjerg O, et al. Analytical goals for the acceptance of common reference intervals for laboratories throughout a geographical area. Scand J Clin Lab Invest 1988;48:757-64.
- Hyltoft Petersen P, Fraser CG, Westgard JO, Lytken Larsen M. Analytical goal-setting for monitoring patients when two analytical methods are used. Clin Chem 1992;38:2256-60.
- Fraser CG. Desirable performance standards for therapeutic drug monitoring. Clin Chem 1987; 33:387-9.
- Fraser CG, Hyltoft Petersen P. Quality goals in external assessment are best based on biology. Scand J Clin Lab Invest 1993; 53 Supp 212:8-9.
- Thienpont L, Franzini C, Kratochvila J, et al. Analytical quality specifications for reference methods and operating specifications for networks of reference laboratories. Eur J Clin Chem Clin Biochem 1995;33:949-57.
Biographical details - Callum G Fraser
Callum was born in Dundee only a few miles from Ninewells Hospital and Medical School. He was educated in Scotland and graduated BSc and PhD from the University of Aberdeen. Following postdoctoral work with the National Research Council of Canada, he returned to his alma mater as Lecturer in Chemical Pathology. He then worked for 8½ years as Chief Clinical Biochemist at the then new Flinders Medical Centre and as Senior Lecturer [then Associate Professsor] in the Medical School of the Flinders University of South Australia. He returned to Scotland in 1983 and is currently Clinical Director of Biochemical Medicine and Honorary Senior Lecturer in both Universities of Dundee and St Andrews. He has published a book, a number of book chapters, and many papers on setting quality specifications and biological variation. He has organised and executed workshops at many conferences and is committed to education and training in laboratory medicine. He has served on committees of a number of national and international bodies including IUPAC and IFCC. He has been honoured by the ACB Foundation Award, the Roman Travelling Lectureship of the Australasian Association of Clinical Biochemists and the IFCC Travelling Lectureship to Kenya and Uganda.